[Papers][CV][Face Detection] Vision-Language Intelligence: Tasks, Representation Learning, and Large Models 논문 리뷰
Vision-Language Intelligence: Tasks, Representation Learning, and Large Models
이번에 리뷰할 논문은 2022년 발표된 Vision-Language Intelligence: Tasks, Representation Learning, and Large Models
이라는 제목의 서베이 논문입니다.
본 논문은 대략 2021년 초까지의 VLP 모델들에 대한 동향을 다루고 있습니다. 본 포스팅을 읽으시고 이후 모델들에 대해 추가적으로 보시면 좋을 것 같습니다.
목차는 아래와 같습니다.
- Introduction
- Task Specific Problems
- Vision-Language Joint Representations
- Scale up Models and Data
- Future Trends
Introduction
하드웨어의 발전과 빅데이터의 등장으로 딥러닝은 빠르게 발전했습니다. 딥러닝의 세부 분야 중 CV(Computer Vision)에서는 CNN 모델이 대세를 이루며 발전했고, NLP(Natural Language Processing)에서는 RNN 모델이 대세를 이루며 발전했죠. 2017년도에는 ‘Attention is all you need’라는 논문이 발표되며 Transformer 모델이 등장했고, NLP 분야에 더해 CV 분야까지 상당한 영향력을 보이고 있는 중입니다. (사실상 잡아먹고 있다고 해도 될 정도…)
하지만 해당 모델들은 domain-specific하고, 이에 Vision-Language Multi-modal을 다루는 모델들이 등장했습니다. Multi-modal이 필요한 이유는 다음과 같습니다.
- 실세계의 문제들은 대부분 multi-modality에 포함된다.
- Single-modality 문제들 또한 multi-modality로부터 이점을 얻는다.
논문에서는 Vision-Language model의 발전사를 시대 별로 정리합니다.
2014-2018
: 특정한 VL 문제를 풀려는 시도 (Image captioning, VQA, Image-text matching)- Image Captioning: CNN image encoder + RNN text decoder
- VQA: Mapping images and texts into the same latent space and predicting answers from the latent representations
- Image-text matching: Sentence-level 혹은 Token-level로 image와 text의 유사도를 계산
2019-2021
: 잘 라벨링된 VL dataset을 사용하여 joint representation 학습- 대량의 데이터셋을 구하기 어렵다는 한계
- 극복 시도
- Contrastive learning
- Large-scale web-crawled data
2021(CLIP이 등장하면서) - 현재
: 대량의 weakly-labeled dataset과 self-supervised learning을 이용한 VL model pretraining
VL intelligence의 일반적인 목표는 visual representation을 잘 학습하는 것입니다. 그리고 이를 잘 표현한다는 것은 3가지 요건을 필요로 합니다.
Object-level
: Image-Sentence 뿐 아니라, Object-word 사이의 의미도 잘 형성되어야 함(feature가 비슷해야 함)Language-aligned
: Language와 align된 vision feature는 vision task를 푸는데도 더욱 도움이 되어야 함Semantic-rich
: Representation은 doamin 제약 없이 대량의 데이터로 학습되어야 함
그리고 위에서 이야기한 3번째 시대의 VLP 모델들만이 세가지 요건을 모두 충족시키며 발전하고 있습니다.
아래서부터는 각 시대 별로 연구 동향을 살펴봅니다.
Task Specific Problems
여기서는 초기 VL 연구들을 소개합니다. 그리고 여기에는 아래와 같은 세부 분야들이 존재합니다.
논문에서는 Image captioning, VQA, Image-text matching에 대해서 자세히 다루고, 나머지는 간략하게 언급만 하고 넘어갑니다.
이 시대의 특징은 각 task에 적합한 모델들이 독립적으로 발전되었다는 것인데, 발전의 흐름은 각 task 모두 동일하게 3단계로 이루어집니다.
- Global vector representation and simple fusion
- Grid feature representation and cross-modal attention
- Object-centric feature representation and bottom-up top-down attention
A. Image Captioning
Task definition
- Generate a caption for a given image
Methods
-
Conventional method
- 객체를 인식하고 predefined rule에 의해 caption 생성
-
First phase
- Seq2Seq 모델의 text encoder를 image encoder(GoogleNet 등)로 바꾼 Img2Seq 사용
- Image encoder의 마지막 feature vector를 Text decoder의 첫번째 hidden state로 사용
- 한계점: Global feature의 사용 만으로는 특정 객체에 대해 focus하지 못한다.
-
Second phase
- Feature map을 C 크기의 feature vector HxW 개로 생성하여 attention
- 한계점: 동일한 크기의 grid 들은 객체를 제대로 반영하지 못 함
-
Third phase
- Detection model이 제안한 region과 bottom-up and top-down attention(BUTD) 사용
- Further researches
- Attention gate: 어떤 region을 사용하고 사용하지 않을 것인가?
- Sentence template을 먼저 생성하고 텍스트 생성?
Summary
- Visual representation & Language decoding
B. VQA
Task definition
- Given and image-question pair, answer a question based on the image
Methods
-
First phase
- question encoder로 LSTM, image encoder로 VGG 사용
- 미리 정의된 N개의 answer로 classification
- Further researches
- CNN image feature들을 LSTM의 각 cell state에 제공
- question encoding과 answer decoding에 동일한 LSTM 공유
- 한계점: VQA는 특정 영역에만 관련있는 경우가 많아서 global feature는 적절하지 않음
-
Second phase
- SAN(Stacked Attention Network): question-guided attention layer를 query로 image grid와 함께 사용
- 한계점: 동일한 크기의 grid 들은 객체를 제대로 반영하지 못 함
-
Third phase
- Question feature를 query로 image attention 시 사용하는 아이디어는 그대로
- Detection model이 제안한 region과 bottom-up and top-down attention(BUTD) 사용
- Further researches
- Co-attention: Text-guided image attention & image-guided text attention 동시 사용
- Image embedding과 language token을 concat하여 LSTM의 입력으로 사용
Summary
- Obtain a joint representation of image and language (question)
C. Image Text Matching
Task definition
- Given a query in a certain modality (vision or language), it aims to find the semantically closest target from another modality.
Methods
-
First phase
- Image와 Text의 global feature를 encoding하여 embedding
- 한계점: 마찬가지로 global feature는 특정 객체를 가리키기에 적절치 않음
-
Second phase
- Grid image와 Text token을 encoding하여 embedding
- 한계점: 마찬가지로 동일한 크기의 grid 들은 객체를 제대로 반영하지 못 함
-
Third phase
- Detection model이 제안한 region(Image fragment)과 Dependency parsing을 이용해 생성한 sentence fragment를 cross-modality embedding space에 mapping
- Further researches
- Context-modulated attention / Dual-attention: Image와 Text 모두에 나타난 instance 활용
- Cross-attention: Image와 Text를 모두 서로의 attention query로 사용
Summary
- Map image and text into a shared embedding space and then calculate their similarity
D. Other Tasks
- Text-to-Image Generation
- Given a piece of text, generate an image containing the content of the text
- Visual Dialog
- Given an image, a dialog history, and a question about the image, answer the question
- Visual Reasoning
- VQA와 유사
- 이미지를 좀 더 깊게 이해해야 하는 진보된 task
- 주로 이미지에 있는 객체, 질문의 구조 등에 대한 적절한 annotation이 주어짐
- Visual Entailment
- Given and image and a text, decide whether the image semantically entails the input text
- Pharse Grounding and Reference Expression Comprehension
- Output bounding box corresponding to the text
- Pharse grounding: Text가 set of phrases
- Reference expression comprehension: Text가 expression
정리하면, 이 시대에는 특정 task를 위한 개별적인 모델들이 연구되었습니다.
Vision-Language Joint Representations
빅데이터의 출현과 하드웨어(GPU, TPU 등)의 발전으로 인해 large scale pre-training이 가능해졌고, 이는 다양한 down-streaming task에 있어 성능의 향상을 불러왔습니다.
VLP 모델들은 기본적으로 Language model 들의 구조와 학습 방식을 따릅니다. 예를 들어 아래 그림은 BERT 모델과 image-text cross-modal 을 위한 naive한 형태의 BERT입니다.
최근 Language model의 특징으로는 Attention mechanism을 사용한다는 것과 MLM(Masked Language Model)의 학습 방식을 이용한다는 것입니다.
VLP 모델은 아래 세 가지 주요 component를 가집니다.
- visual embedding (VE)
- textual embedding (TE)
- modality fusion (MF)
VE와 TE는 각각 image, text로 pretrained된 모델을 사용하고, MF는 VE와 TE에서 추출된 feature를 어떻게 잘 합칠지(align시킬지)에 대한 것입니다.
그리고 위에서 말했듯, 여기에서 중요한 세 가지 목적은 아래와 같습니다.
Object-level
: Image-Sentence 뿐 아니라, Object-word 사이의 의미도 잘 형성되어야 함(feature가 비슷해야 함)Language-aligned
: Language와 align된 vision feature는 vision task를 푸는데도 더욱 도움이 되어야 함Semantic-rich
: Representation은 doamin 제약 없이 대량의 데이터로 학습되어야 함
위 특징들로 인해 VLP는 적은 데이터셋을 가지는 down streaming task에서도 수준급의 성능을 낼 수 있도록 합니다.
A. Why Pre-training is needed?
딥러닝은 근본적으로 통계에 기반한 접근입니다. 딥러닝 모델은 학습 데이터의 분포를 익히고, 그 분포를 바탕으로 unseen data에 대한 예측을 수행합니다.
학습을 진행할 때에는 학습 데이터의 loss를 줄이는 것이 unseen data에서의 loss를 줄이는 것이라고 믿고, 학습 데이터에서 학습을 진행합니다.
이는 매우 통계학적인 접근이며, 이 논리가 성립하기 위해서는 아래 두 가지 조건이 필요합니다.
- 데이터가 충분히 많아야 함
- 데이터가 independent and identically distributed (i.i.d) sample이어야 함
하지만 당연하게도, 대부분의 경우에 1의 조건을 만족하지 못 합니다. 따라서 학습 데이터로부터 예측하지 학습하지 못 한 분포에 해당하는 데이터에 대해서는, 좋지 않은 성능을 보이게 됩니다.
Pre-training은 바로 이를 완화하는 역할을 합니다. 충분한 양의 데이터로 학습된 모델을 제공함으로써, 이후에 적은 양의 데이터로 학습되는 down-streaming task에서도 데이터의 분포를 어느정도 잘 예측하도록 합니다.
B. Modality Embedding
Text feature와 image feature는 기본적으로 다르기 때문에, 두 feature를 각각 추출하여 하나의 feature space에 mapping하는 방식을 이용합니다.
이때 어떤 데이터셋을 사용하느냐와 어떤 하이퍼파라미터 값을 사용하느냐가 VLP 모델의 성능에 영향을 미칩니다.
- Text Tokenization and Embedding: 요즘은 BPE(Byte Pair Encoding)를 사용하여 text를 tokenization 한 뒤 embedding합니다.
- Visual Tokenization and Embedding: Tokenization 방법에 따라 embedding 방식이 결정됩니다.
- Grid features: CNN에서 추출된 feature map을 일정한 grid로 나누어 사용합니다. 이것의 장점은 pretrained object detector가 필요없다는 것이고, 배경에 대한 정보가 사용될 수 있다는 것입니다.
- Region features: Pretrained object detector (Faster R-CNN trained by Visual Genome(VG) dataset)를 사용하여 영역을 추출합니다. 여기서 추출된 영역은 bounding box, object tag, RoI feature의 3개의 특징을 가집니다.
- Patch features: Grid feature와 다른 점은 image 자체를 일정 크기의 patch로 나눈 뒤 linear projection을 통해 바로 embedding됩니다.
C. Modality Fusion
MF에는 두 가지 방법이 있습니다.
- Dual stream modeling
- Text와 Image 각각에서 embedding된 feature를 하나의 joint representation feature space로 mapping합니다.
- Text와 Image 각각에 적절한 다른 구조의 encoder를 사용할 수 있다는 장점이 있습니다.
- 그러나 다른 feature를 동일한 공간에 mapping 해주는 과정을 explicit하게 해주어야 합니다.
- Single stream modeling
- Text와 Image feature를 동시에 사용해서 Unified encoder에 입력합니다.
- 사람의 개입 없이 모델이 implicit하게 joint representation을 학습합니다.
- 현재 대부분의 VLP 모델이 이 방식을 사용합니다.
D. Training
VLP 모델은 self-supervised learning loss를 사용하며, 크게 3가지 학습 방법들을 사용합니다.
-
Image Text Matching (ITM)
-
텍스트와 이미지 간 특징의 유사성을 학습하기 위한 방법
-
W (sequence of language tokens)와 V (visual contents)가 있다고 할 때, ITM 문제는 binary classification 문제로 치환될 수 있습니다.
-
-
Masked Language Modeling (MLM)
- 단어들 간 관계를 학습하기 위햔 방법
- W\i (i-th word가 빠진 sentence)와 V (visual contents)가 있다고 할 때 빠진 단어를 맞추는 문제
- masking되는 것을 subword가 아니라, 하나의 word가 되어야 함. 이는 subword는 주변 subword들로부터 쉽게 예측이 가능하기 때문.
- 더 발전된 Knowledge Masked Language Modeling으로 phrase-level masking, entity-lavel masking 등도 있음
-
Masked Visual Modeling (MVM)
-
이미지 내의 내용들 간 관계를 학습하기 위한 방법
-
MLM과 달리 MVM에서는 language modality 없이도 visual content를 예측하는 것이 가능해서, 이 괴리를 줄이기 위해 다양한 학습 방법들이 제안됨
-
Masked Region Prediction (MRP): 모델이 예측한 feature와 detector가 추출한 feature 간 l2 norm 측정
-
Masked Region Classification (MRC): 모델이 분류한 클래스와 detector가 분류한 클래스 간 CE 측정
-
Masked Region Classification with KL-Divergence: MRC에서 detector가 분류한 클래스가 무조건 맞다고 보장할 수 없기 때문에, 마지막 출력인 softmax 값 사이의 KL-divergence 값을 측정
-
Masked Visual Modeling with Visual Dictionary (MVMVD): Region을 visual dictionary(VD)에 대응하여 VD token을 예측하는 문제로 치환
- SOHO 논문에서는 이웃한 image grid들은 같은 VD에 매핑될 가능성이 높아서, masking 시 같은 VD로 매핑되는 모든 visual content들을 masking함
-
-
Inter-modal fusion을 encourage하기 위해 한 번에 한 가지 학습 방법만을 사용해 학습을 진행합니다.
E. Landscape of General Pre-training Studies
Scale up Models and Data
앞선 시대의 모델들은 object-level representation에 집중하여 좋은 cross-modal alignment를 달성하려 했습니다. 하지만 여기에는 데이터셋이 아주 잘 라벨링되어야 한다는 큰 제약 사항이 있습니다. 따라서, 이 때의 모델들은 대량의 데이터셋을 사용하지 못했습니다.
세번째 시대에 들어서며, 모델들은 richer semantic과 stronger generalization capability를 얻기 위해 weakly-labeled dataset들을 적극 활용하였습니다.
그 예시로 Visual understanding의 CLIP, visual gneration의 DALL-E가 있으며, 각각 400M개와 250M개의 large-scale weakly labeled dataset들을 학습에 사용하였습니다.
A. Visual Understanding
CLIP
의 핵심 아이디어는 학습 방법에 있습니다.
CLIP에서는 image-text pair를 학습하기 위해 N개의 image-text pair가 주어졌을 때 총 NxN 가지의 가능한 pair들 중 어느 것이 matched pair이고 unmatched pair인지 학습합니다. 이는 곧 image와 text가 잘 align되는 NxN matrix를 학습한다는 것으로 이해할 수 있습니다.
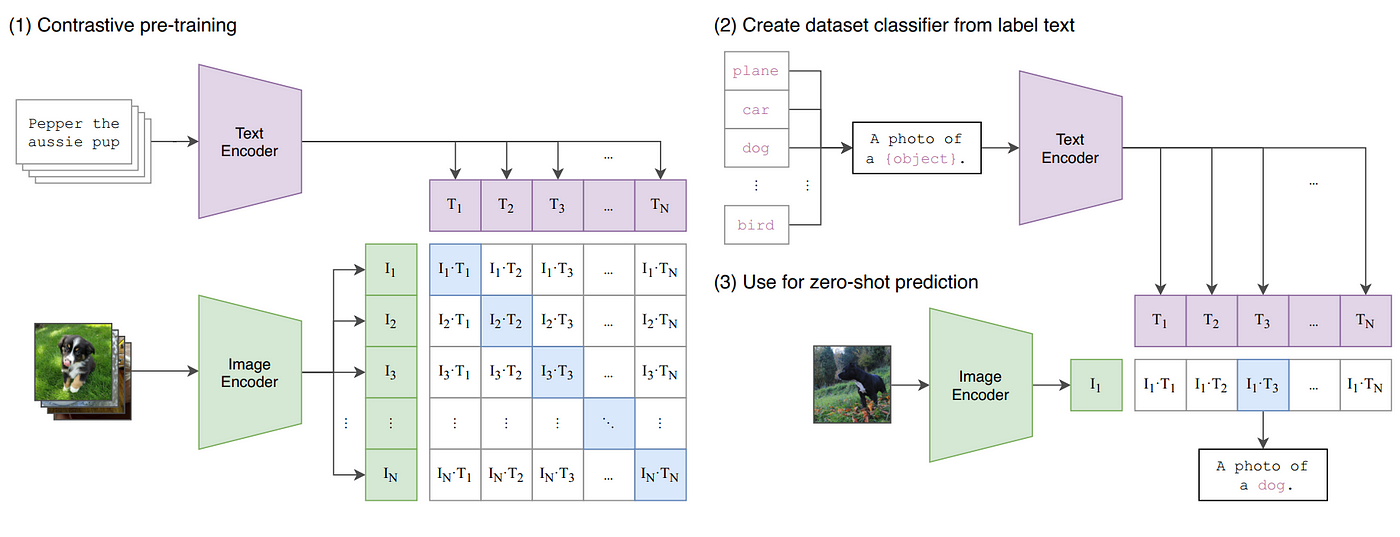 |
이후 발표된 ALIGN
은 CLIP과 유사하게 dual encoder model과 contrastive loss를 사용하였고, 대신에 1.8B 크기의 더 대량의 데이터셋을 사용함으로써 많은 zero-shot visual task에서 CLIP을 뛰어넘는 성능을 보였습니다. 이로부터 더 큰 데이터셋이 더 나은 성능을 불러온다는 것을 검증하였습니다.
SimVLM
은 조금 다른 VL pre-training 방식을 사용하며 이에 준하는 성능을 냈습니다. (Simple prefix language modeling objective to predict the next token in an autoregressive way)
Florence
는 앞선 모델들이 coarse (image-level) representation과 static (image) data를 사용한 것에서 더 나아가, fine-grained (object-level) representation과 dynamic (video) data를 사용하여 모델을 학습했습니다. 이를 위하여 image encoder에 Dynamic Head를 추가하였고, extra object detection dataset으로 학습하였습니다.
B. Visual Generation
DALL-E
는 image를 discrete variational autoencoder (dVAE)를 사용하여 visual token으로 매핑(codebook)합니다. 이로써 (text, image) pair를 하나의 single stream data로 취급할 수 있게 되었습니다.
DALL-E는 4가지 측면(creating anthropomorphized versions of animals and objects, combining unrelated concepts, rendering text, and applying a transformation to existing images)에서 뛰어난 모습을 보였습니다.
GOVIDA
는 text-video generation을 위한 모델로, video의 각 frame을 tokenize하여 text와 concatenate함으로써 마찬가지로 하나의 stream으로 모델에 제공됩니다.
이후 Wu et al. (2021b)
라는 논문에서는 3D Trensformer를 이용해 text(1D), images(2D), video(3D)를 모두 encoding 가능한 형태의 모델 구조를 제안하였습니다.
Future Trends
앞으로의 연구 트렌드는 크게 아래 세가지로 나타낼 수 있습니다.
- Toward Modality Cooperation
- 특정 modality의 성능을 높이기 위해 다른 modality의 데이터를 이용하거나, multi-modality 성능을 높이기 위해 single-modality 데이터를 이용하는 것에 대한 연구
- Language 모델의 성능을 높이기 위해 text-image 로 학습된 모델을 활용하는 것 등
- Toward General Unified-Modality
- 다양한 modality의 feature들을 general representation으로 unify할 수 있는 방법에 대한 연구
- UNIMO는 single-modal/multi-modal, understanding/generation을 모두 포함하는 unified pre-training model을 제안
- VL + Knowledge
- VLP 모델에 extra knowledge를 더하는 연구
- Masking 시 word-level masking 뿐 아니라 phrase-level, entity-level masking을 함께 사용하여 모델을 더욱 고차원적으로 학습시키는 방법
- 이용하고 싶은 knowledge로 특정 모델을 먼저 학습시킨 뒤, VLP 모델 학습 시 knowledge embedding과의 auxiliary loss를 통해 knowledge를 학습시키는 방법 등
이를 위하여 앞으로의 VLP 모델들은 더 많은 데이터셋, 더 큰 크기로 진화할 것으로 보입니다.
논문에 대한 내용은 여기까지입니다. 아래부터는 개인적으로 새롭게 알고 느끼게 된 부분들을 정리하는 부분입니다.
새롭게 알게 된 것들
Vocabulary
Vocabulary | meanings |
---|---|
benefit from | ~로부터 이점을 얻다 |
axiom | 공리, 격언, 자명한 이치 |
prosody | 작시법 |
tailor/be tailored for | 재단사/맞춤형의 |
surge | 큰 파도, 동요; 급등하다 |
urge | 충고하다 |
granularity | 세분성 |
aforementioned | 앞서 말한 |
encompass | 에워싸다 |
trajectory | 궤도; 사선 |
encompass | 에워싸다 |
propensity | 경향 |
tailored | 맞춤형의 |
extrapolate | 외삽하다 |
corpora | 말뭉치 |
Leave a comment